
On November 20, we held a live workshop to help logistics service providers prepare to build more data-driven supply chains in 2025.
Why is this a priority now? We’re seeing a major shift in customer expectations: from “what happened?” to “what will happen and how can I improve?” Though this isn’t a new ask from your customers, the capabilities and tools available to you are making these predictive questions actually answerable.
Brian Glick, CEO of Chain.io, and Christian van Eeden, VP of Transformation Architecture at Hellmann Worldwide Logistics, discussed the expectations of LSPs from retail customers, how to experiment with AI and make the most of the analytics opportunities it offers, and even shared a reference architecture that your IT and data teams can use to model your own tech stack.
The biggest takeaway beyond AI and data architectures? LSPs and Shippers should be leaning on each other more as strategic partners. Each has detailed, vertical specific knowledge that can help the other perform better and grow.
Check out the full recap of yesterday’s session below. If you’d rather listen along, you can watch the full recording here:
Watch the recordingThe Evolution of Supply Chain Data Expectations
Things have (obviously) changed in the last 20 years of supply chain tech.
In 2004, answering questions about freight shipped last month was sufficient, but the pandemic marked a major shift for many LSPs as customers began asking questions about the status of their shipments in real-time. In the midst of disruptions and supply chain chaos, LSPs had to adapt their technical capabilities to answer that question - and struggled to find the answers through dozens of phone calls, emails, and buried in spreadsheets.
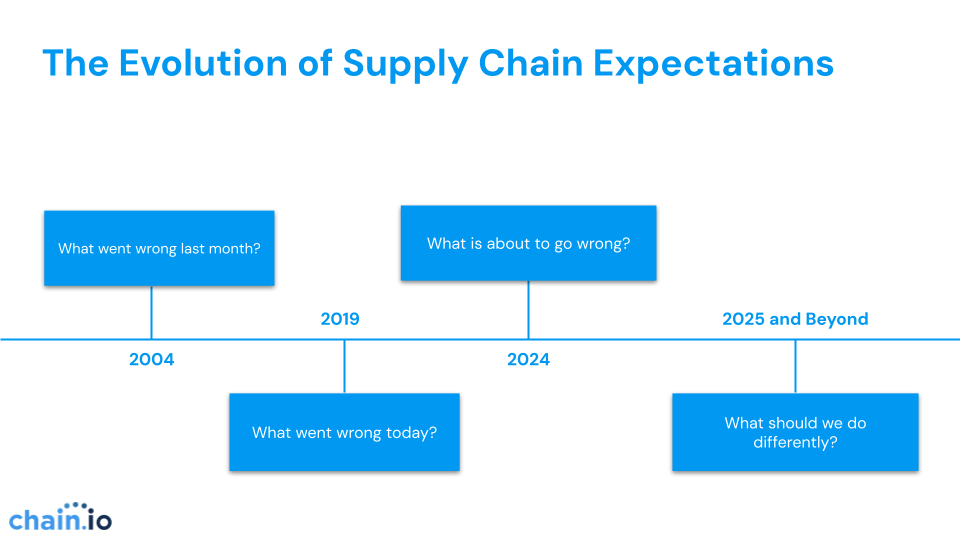
While we’re moving away from that reality and real-time data sharing has become more attainable, expectations are shifting yet again. Now, your customers want to know what is about to go wrong and what they should do differently to avoid risks and problems in the future.
To answer their questions, LSPs and shippers have to get deeper into their supply chain data earlier. By working together with shippers, suppliers, and tech providers, you can get the right information to answer the “what should I do differently” questions you’ll be faced with.

From left to right, this matrix models expectations of departments vs. the whole enterprise. From top to bottom, it models what is perceived knowledge (based on analysis) to what is pure fact (based on a single data point.)
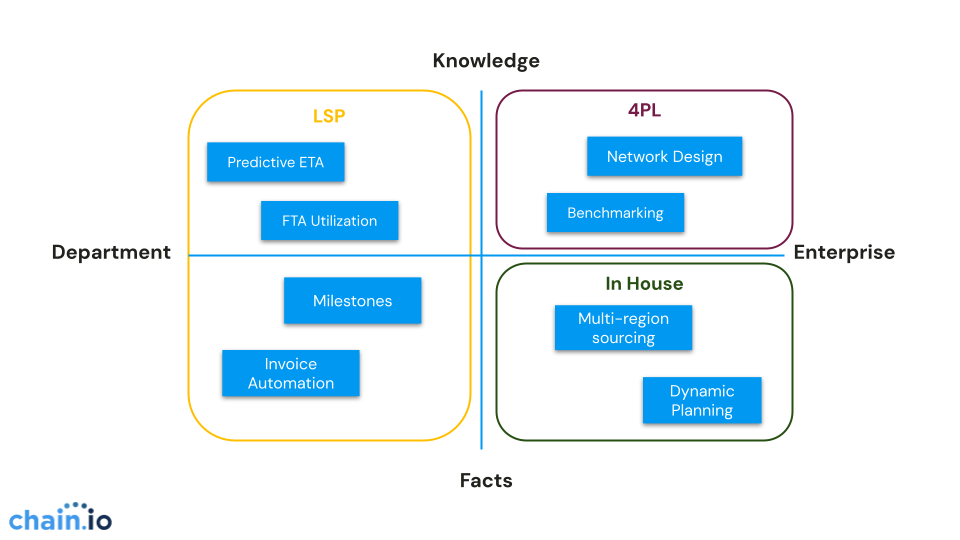
Captured in this matrix are the teams that can provide insight into each decision:
- LSPs can share data-based facts with supply chain departments like invoices and milestone updates, as well as deeper insights like predictive ETAs and FTA utilizations.
- 4PLs can give even more insights to the entire organization like advice on network design or expectations for future freight movement.
- Shipper’s in-house supply chain teams are then responsible for company wide, fact based details like regional sourcing decisions and dynamic planning.
Having enough information about past performance, risks, and ongoing challenges isn’t enough to make the decisions or answer the questions that are asked of your team. There is too much data to sift through manually, too many trends to analyze, and not enough time or resources to accomplish it without leveraging some advanced analytics and AI tools.
How does AI fit in?
AI Definitions for Supply Chain Context
Generative AI: generates new information based on prompts
- Example prompt: “Based on my company’s activities over the past year and where the economy is going, make an RFP for the best lanes and volumes for next year”
Predictive AI: uses past patterns to make future predictions
- Example prompt: “Here’s a million arrival and departure dates. How likely is it that this shipment will arrive on or before May 5?”
The Real Value of AI for Supply Chains
When we talk about leveraging AI tools, you shouldn’t select a tool just because it's “AI Powered.” This label gets attached to so many new piece sof supply chain tech, but AI alone doesn’t make a tool useful.
What you should really care about from an AI tool is the results that it returns and how it helps you do your work faster. There are hundreds of thousands of data points you can input in an AI model to analyze past trends, uncover new opportunities, and make future predictions, but the results you receive are only as good as your inputs.
When you start testing and using AI tools for your business, make sure that the data you’re using is accurate and in a standard format. You want to ensure that each AI response helps your business either:
- Answer customer questions faster and improve their overall experience
- Find ways to increase efficiency and cut costs
- Realize new opportunities for your business
- Share recommendations for improving your customers business
LSP Strengths
As an LSP, your biggest advantage to your customers is the vast amount of knowledge you have about efficient freight movement and market conditions. With all of this knowledge, you can excel at:
- predicting ETA
- freight audit
- trade compliance risk
- origin management automation
- tariff engineering prompts
- supplier survey management
Your broad industry knowledge positions you well to become a strategic advisor and trusted partner to your customers. You may have advice on where to build warehouses, where to adjust workforces, where to take advantage of service centers, upcoming regulation changes, and what’s happening next in the freight market.
There’s a strong chance you know more about customer supply chains than they do, so as you listen to their needs, share recommendations and advice for operating more efficiently. AI tools can help you do this better and at scale.
Smaller LSPs and freight forwarders can use their specific focuses as an advantage to their customers. If you operate in a specific lane or with a specific shipment type, you can share very specific advice and knowledge to boost your customer’s happiness.
Shipper Strengths
Your shipper customers have very specific context about their business which positions them well to answer questions like these internally:
- Where should we source?
- How do we balance risk and complexity?
- How do we achieve optimal landed cost?
- Will we move more or less freight next year?
As an LSP, you support your customers by automating shipment data to their systems so they have all of the information needed to answer these questions.
Large shipper customers often try to manage supply chains alone. They aren’t always talking and engaging with their service providers. You can encourage this collaboration in each interaction you have with customers, whether it's sharing advice in your email responses, or scheduling a lunch and learn to educate customers about changes and trends they should be aware of.
A Reference Data Architecture
On top of everything you do for customers today, LSPs need to be prepared to provide more raw data and facts to customers. Shippers are expecting real-time access to this information, and automating updates between your systems is the first step in this process. This transparency is the only way you can effectively solve problems and answer the “what will happen” questions.
Determining whether you share only raw data (the facts), or summarized learnings (the knowledge) with your shipper customers depends on their size and capabilities.
- Small to mid-size shippers want knowledge from you. They likely don’t have an AI or Data team to analyze all of the information you share.
- Large shippers will want all of the data you have that relates to their business. They will leverage data teams and AI tools to analyze the vast amounts of data to find the answers they need.
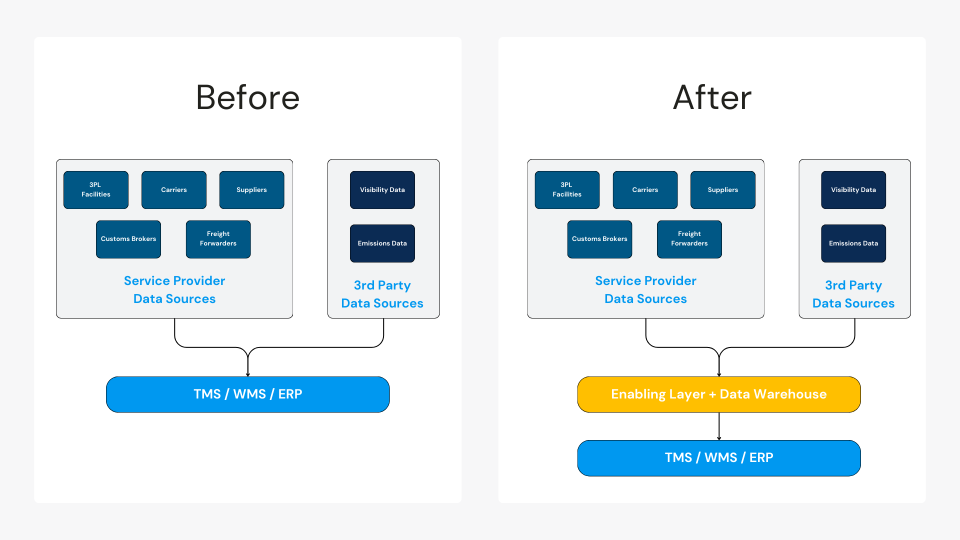
To support data sharing and transparency with customers, you’ll have to have the right data architecture in place.
“Before” is how many LSPs have previously thought of data ecosystems, and how shipper and LSPs integrations have been structured. Many LSPs are still using this framework, with a TMS at the core of their ecosystem.
Some challenges with this:
- You can only work in the formats these systems are built
- Analysis becomes really difficult
- Your TMS receives data in all formats and naming conventions - likely not a lot of standardization
Future data ecosystems are shifting to to have an enabling layer and data warehouse at their core. Feeding all raw data into the enabling or integration layer ensures that the data sent to your data warehouse, and ultimately TMS and customer systems, is sent in one standard, clean, and accurate format.
This setup gives you the information you need to answer customer questions faster, especially as you adopt AI and analytics tools into your tech stack.
How does Chain.io help?
The Chain.io platform is designed to be your enabling layer and powers data-driven supply chains for logistics service providers and supply chain teams. Our platform connects your data sources (customers, third-party systems, suppliers, and internal systems), normalizes the data, and makes it accessible to your entire organization and ready to share.
If you want to dig deeper into this topic, check out the full recording below, or schedule some time to meet with one of our supply chain experts.
Watch the RecordingBook a Demo